Research team
Prof. John Wanjala Makokha1 _principal investigator; Dr. Martha Muthoni Konje2 _co-PI. Other team members include: Dr. Anselemo Peters Ikoha, Dr. Jonathan Mutonyi, Dr. Alice Nambiro, Dr. Nelly Masayi, Mr. Victor Okello, Mr. Collins Majengo and Mr. Peter Barasa. Postgraduate students include; Eric Sifuna and Eve Wamasebu who are PhD and Masters students respectively under the project sponsorship.
Overview
The research is as a product of the partnership between RUFORUM RAINCA and Kibabii university through a competitive grant. The year-long study aimed at establishing the Use of Smart Technology to Predict Climate Change Effects on Terrestrial Plants Diversity and Conservation for Sustainable Livelihood in eight counties in North-Rift Region, in Kenya. The targeted counties of; Turkana, Trans-Nzoia, West Pokot, Uasin Gishu, Nandi, Baringo, Elgeyo Marakwet and Samburu, with the research running from April 2023 to May 2024, with a goal find out communities’ understanding of climate change, and the effects of climate change.
The data was captured from respondents from five counties in the republic of Kenya namely Trans-Nzoia, West-Pokot, Nandi, Turkana and Samburu. A total of 50 questionnaires were distributed to 10 respondents in each of the five counties that were visited. The study recorded a 92% return rate, was noted a positive return.
Results
Participants’ characteristics such as age, gender, occupation, education levels were captured by the data collection tools. Analysis indicated that the youthful population between the ages of 26-45 formed the majority of the respondents at 56.52% with the population above 46 being 56.52%. The gender of the respondents were 33 males and females were 13 that is 71.74% and 28.26% respectively. Majority of the respondents had acquired basic education having both primary and secondary qualifications at 63.04% while tertiary and above had 2.17%; while those without any formal schooling was at 34.78%. The respondents indicated to engaged in occupations that include; farmer, manager, student, teacher, broker of animals, community forest scout, business lady and pastoralist. Farmers were a majority at 80.44% while manager, student, broker of animals, community forest scout and pastoralist each having the lowest at 2.17%.
The study established that the communities in Kenya have adequate knowledge of climate change, and their causes and effects. On the medium of accessing information, sampled population indicated that radio was the most common source of information on climate change while indigenous knowledge came in second, thus highlighting on the pivotal role played by traditional sources of knowledge in the study area.
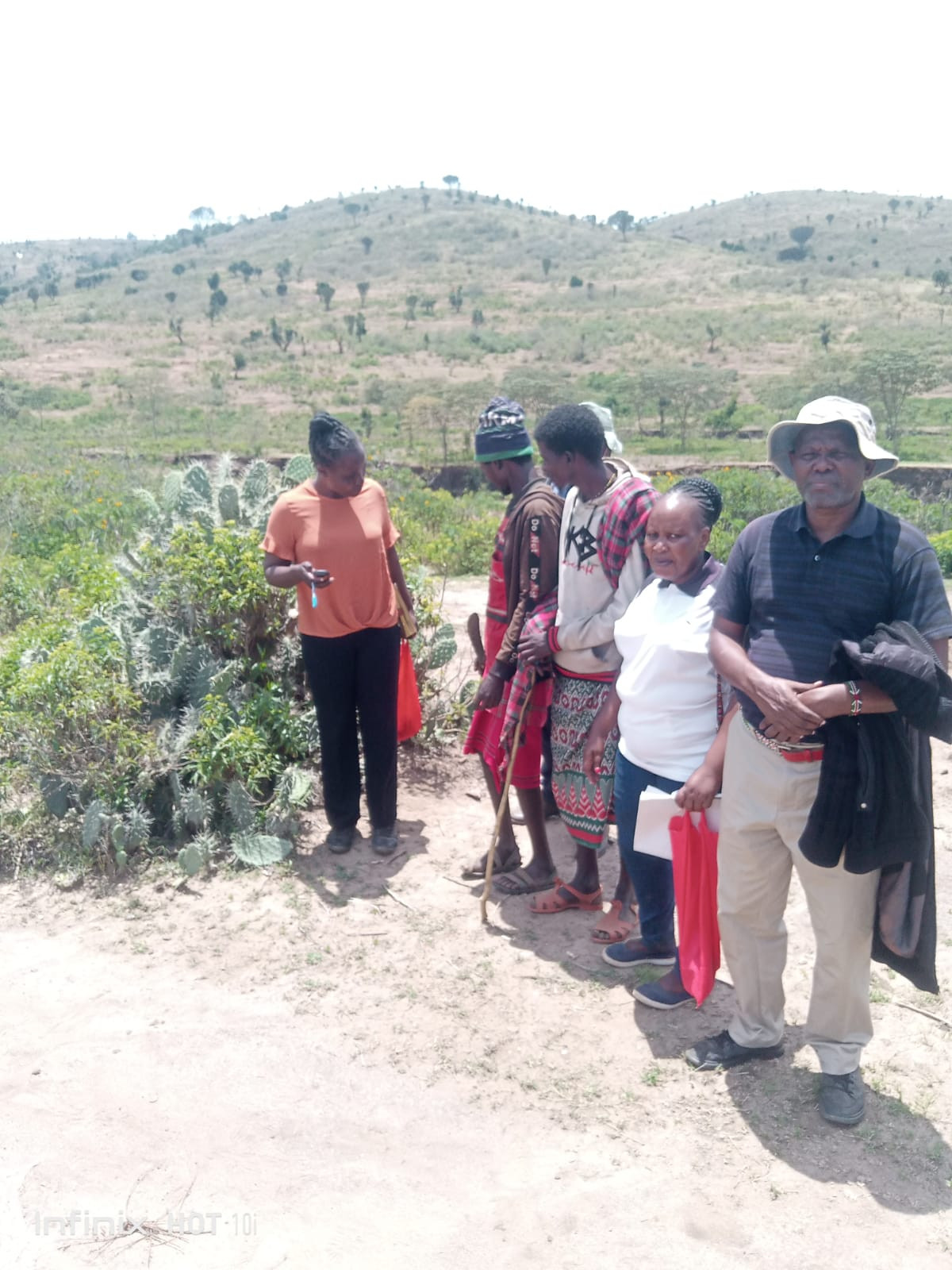
A considerable number f participants blamed deforestation as the major cause of climate change, with changes in weather patterns also featuring prominently. Many agreed that the effects of climate change were being felt with a majority indicating that rainfall had decreased amid increase in temperatures, prolonged droughts and an increase in destructive winds. The study also noted other effects of climate change to be; low food production across the target counites, as well as scarcity in animal feeds.
to The study established that residents of the participating counties believe that afforestation is a reliable method of mitigating climate change and that the communities and government are actively involved in the afforestation exercises, whereas the national government plays an important role of creating awareness to the public on climate change.
This research went further to conduct plant identification in the selected sites of the project’s study area
Through the plant identification, a total of 139 distinct plant species belonging to 46 families, 119 species were indigenous while 20 species were exotic. This distribution of species implies that study area was a near natural ecosystem hence restoration practices should consider more of the indigenous species than the exotic ones. The study further identified and disintegrated the most dominant plants in the selected places according to the species and families using local names and the same given in scientific names [brackets] as follows.. Kimwan (fabaceae dominant), 28 species 16 families Mtemburi (even spread), 9 species 9 families Chepkomngony chemater (Rutaceae, rosaceae, euphorbiacea), 10 species 5 families .
Further, the plant identification process was able to group the plants according to their geographical locations as follows. From Sagumai Kirisa Forest, in Samburu, there were 45 Species 29 Familiesof Eurphorbiaceae Lamiaceae, Samburu Loturo Shrub Land (Fabaceae)13 Species 8 Families, Turkana Shrubland 6 Species 2 Families with Salvadora Sp Dominant (Salvadaraceae), from Turkana Riverrine, 20 Species 12 Families (Poaceae), Kamatira Forest 61 Species 30 Families (Fabaceae), West Pokot Shrubland 3 Families, Chorlim-Endebess Grass Land 33 Speceis 17 Families (Fabaceae + Verbenaceae), Kaptega Chepchoina 40 species 24 families (Asteraceae/compositae)
Furthermore, a number of invasive species such as; Prosopis juliflora, cuscuta, Lantana camara, and Ricinus communis were cited. Tree species that have high yielding timber from the same location including; Olea capensis, Podocarpus latifolia and Juniperus procera were also identified.
The research observes that given the rural setup of the research site, the communities should be encouraged to plant species like Maesopsis eminii, and pollinator attractant species such as Leonotis nepetifolia.. Moreover, a number of plants like Jatropha curcas and Catha edulis that have the ability to generate alternative income for the should also be encouraged and embraced by the relevant authorities like the Kenya Forestry Services and the county governments . Several medicinal plants were cited and should be incorporated in the pharmacopeia.
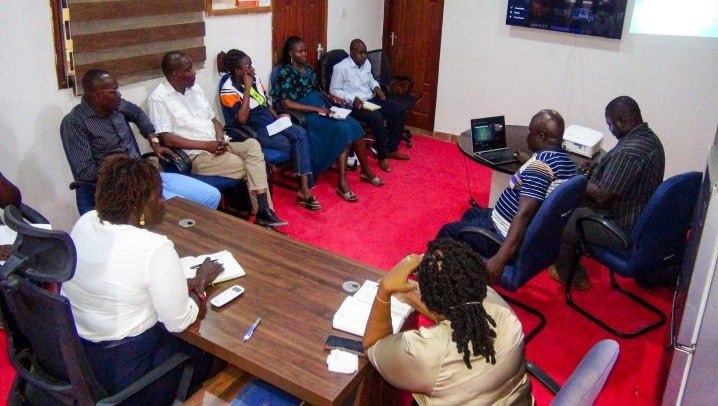
Outputs of the project
The output of the project was modelling the North Rift Weather Prediction System (NRWPS). The NRWPS system was developed with two key components: Normalized Difference Vegetation Index (NDVI) and Bare Soil Index (BSI) prediction. This advancement creates a comprehensive environmental monitoring tool the North Rift region of Kenya and could be adopted in other regions globally.
The NRWPS is an advanced, data-driven environmental forecasting tool designed for eight counties in the North Rift region: Trans-Nzoia, Uasin Gishu, Nandi, Turkana, Baringo, West Pokot, Samburu, and Elgeyo Marakwet. The system is built with Key features that include (a) Multiple prediction models: ARIMA, SARIMAX, and Prophet for weather forecasting, (b) Seasonality analysis for rainfall and temperature patterns, (c) Region-specific predictions considering unique geographical characteristics, (d) Short-term and long-term forecasting capabilities, (e) NDVI prediction for vegetation health assessment using XGBOOST algorithm, (f) Bare Soil Index prediction using XGBoost algorithm. NDVI provides crucial information on vegetation health and density, useful for crop monitoring and drought assessment while BSI offers insights into soil exposure levels, critical for agricultural planning and land degradation monitoring. These indices, combined with weather forecasting, create a powerful tool for comprehensive environmental monitoring and agricultural support.
It is expected that the developed NRWPS shall be subjected to rigorous testing and validation processes which could include (a) Historical data validation against 30-year dataset for weather patterns (b) RMSE analysis for regional weather predictions (c) Cross-validation to ensure model robustness (d)Expert review by local meteorologists, agronomists, and climate scientists in Turkana County.
The NDVI and BSI prediction models, implemented using XGBoost, have demonstrated high accuracy for Mean Absolute Error (MAE): 0.02415710282485725, Mean Squared Error (MSE): 0.0015260731719066503 and R-squared: 0.9445175151888744. These metrics indicate exceptional performance, with the model explaining 94.45% of the variability in the data. The low MAE and MSE values further confirm the model’s precision in predicting both NDVI and BSI.
Notably, the NRWPS has significant scaling potential on; (a) Infrastructure expansion to handle increased computational demands (b) Integration of additional data sources, including high-resolution satellite imagery for NDVI and BSI calculations (c) Geographical expansion to cover neighboring regions (d) Continuous model refinement and XGBoost hyperparameter tuning (e) API development for integration with other systems and applications (f) Automated NDVI and BSI mapping and trend analysis implementation (g) Integration with IoT devices for ground-level data collection
Functionally, the NRWPS is designed for broad community adoption through (a) User-friendly mobile application development (b) Agricultural integration:NDVI and BSI forecasts for informed decision-making on crop health, soil management, and irrigation planning, Alerts for potential crop stress or soil degradation based on NDVI and BSI trends (c) Collaboration with disaster management authorities for early warnings on droughts and land degradation (d) Educational programs on interpreting NDVI and BSI for agricultural and environmental management (e) User feedback mechanism for continuous improvement (f) Interface and predictions available in local languages (g) Customized alerts including NDVI and BSI thresholds (h) Land management support:- (i) Recommendations for crop selection and soil conservation based on NDVI and BSI predictions and collaboration with agricultural agencies on sustainable farming practices (i) Research partnerships with academic institutions for further environmental studies
Conclusions from the Research
In conclusion, the integration of NDVI and BSI prediction using XGBoost significantly enhances the NRWPS’s capability to support agricultural practices and land management in the North Rift region. The high accuracy of the prediction models provides reliable information about vegetation health and soil exposure, crucial for making informed decisions about crop management, irrigation planning, and land degradation monitoring.
By combining weather forecasting with NDVI and BSI predictions, the NRWPS offers a holistic approach to environmental monitoring and agricultural support. This comprehensive tool has the potential to improve agricultural productivity, enhance soil conservation efforts, and support sustainable land management practices in the North Rift region of Kenya.
The successful implementation and high accuracy of the NDVI and BSI prediction models demonstrate the NRWPS’s potential for further enhancements and its value to the local community. Continued development, scaling, and community engagement will be key to maximizing the system’s impact on regional agriculture and environmental management practices.
This innovative system represents a significant step forward in precision agriculture and environmental monitoring for the North Rift region, with the potential to contribute to food security, sustainable land use and climate change adaptation strategies.